

In an observational study such as the Framingham Heart Study, it might be of interest to compare mean blood pressure or mean cholesterol levels in persons who are underweight, normal weight, overweight and obese.
#ANOVA XLSTAT TRIAL#
In a clinical trial to evaluate a new medication for asthma, investigators might compare an experimental medication to a placebo and to a standard treatment (i.e., a medication currently being used). For example, in some clinical trials there are more than two comparison groups. The specific test considered here is called analysis of variance (ANOVA) and is a test of hypothesis that is appropriate to compare means of a continuous variable in two or more independent comparison groups. The hypothesis is based on available information and the investigator's belief about the population parameters. This module will continue the discussion of hypothesis testing, where a specific statement or hypothesis is generated about a population parameter, and sample statistics are used to assess the likelihood that the hypothesis is true. Roy’s test: This table provides the results of Roy’s greatest root test which tests the hypothesis of equality of the mean vectors for the different levels.Hypothesis Testing - Analysis of Variance (ANOVA)īoston University School of Public Health Pillai’s test: This table provides the results of Pillai’s trace test, which tests the hypothesis of equality of the mean vectors for the the different levels. Hotelling-Lawley test: This table provides the results of Hotelling-Lawley trace test, which tests the hypothesis of equality of the mean vectors for the different levels. Wilks’ test (Rao’s approximation): This table provides the results of Wilks' Lambda test which tests the hypothesis of equality of the mean vectors for the different levels. SSCP matrices: These tables are displayed to give a general view of the effects of the factors and interactions between factors. Means of level factor: This table provides for each level factor and quantitative variable the mean value. For qualitative explanatory variables, the names of the various categories are displayed together with their respective frequencies. The number of observations, missing values, the number of non-missing values, the mean and the standard deviation (unbiased) are displayed for the dependent variables (in blue) and the quantitative explanatory variables. Summary statistics: The tables of descriptive statistics show the simple statistics for all the variables selected. When the numbers of all categories for one of the combinations of factors are not equal, then the MANOVA is said to be unbalanced. We speak about balanced MANOVA when the numbers of categories are equal for all combinations of factors. And a potential correlation between response variables is more likely to be detected when these variables are studied together, as is the case with a MANOVA. Instead, the MANOVA is sensitive to both the difference of averages between levels of factors and the covariation between explanatory variables. The potential covariation between response variables is not taken into account with several ANOVAs. MANOVA also enables the simultaneous tests of all hypotheses tested by an ANOVA and is more likely to detect differences between levels of factors.įurthermore, the computation of several ANOVAs instead of one MANOVA increases the Type I error, which is the probability that the null hypothesis will be wrongly rejected.
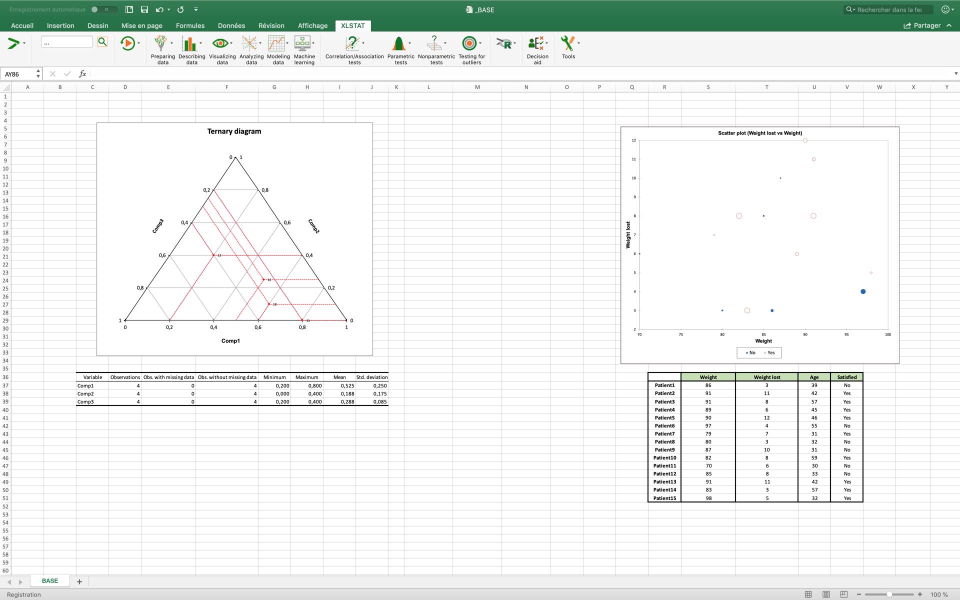
The MANOVA tests the presence of significant differences among combinations of levels of factors on several response variables. The advantage of the MANOVA as opposed to several simultaneous ANOVAs lies in the fact that it takes into account correlations between response variables, which results in a richer use of the information contained in the data. Effects of factors are estimated on a combination of several response variables. With the MANOVA, explanatory variables are often called factors. The main difference comes from the nature of the dependent variables: instead of a single one, we can study many of them. The MANOVA uses the same conceptual framework as the ANOVA. Description of the MANOVA in XLSTAT General description
